The rapid evolution of generative AI technologies has disrupted traditional content creation methods, paving the way for a new era of intelligent content generation. As AI-powered tools like GPT-4 and beyond become more prevalent, production management for generative AI content is becoming increasingly important. In this blog post, we'll discuss the fundamentals of managing AI-generated content production, best practices, and the role of humans in the process.
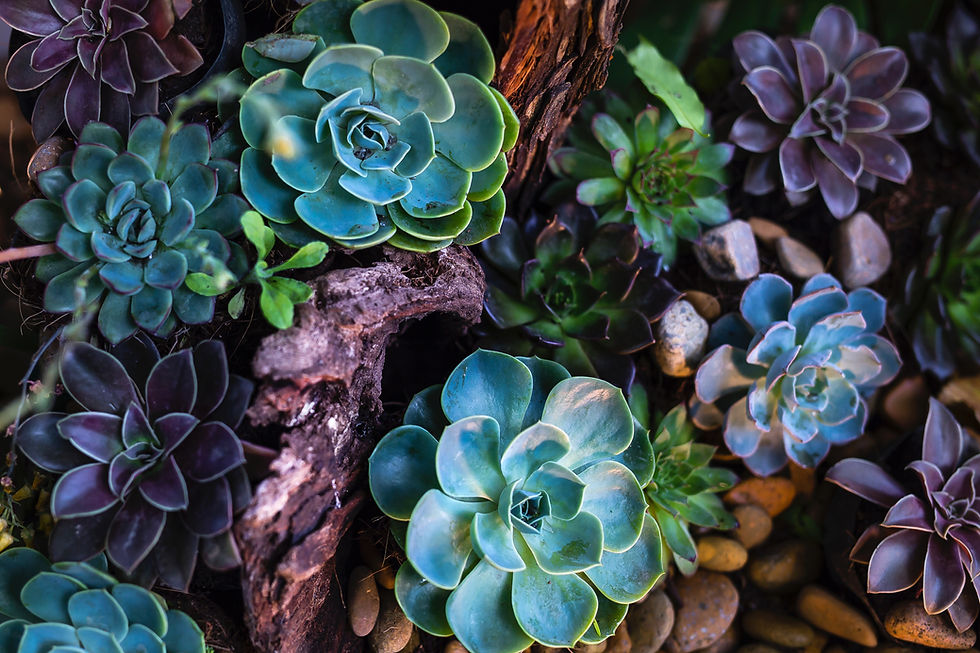
1. Understanding Generative AI Content Production
Generative AI models learn from vast datasets to generate human-like content. These models analyze the context, structure, and style of text to create new content that mimics human language. As a result, AI-generated content can save time and resources, allowing for more efficient content creation.
2. The Importance of Data Management
The quality of generative AI content is heavily influenced by the quality of data used to train the models. Ensuring that the data is diverse, relevant, and representative of the target audience is crucial for producing high-quality content.
Best practices for data management include:
Regularly updating datasets to maintain relevance
Curating data sources to avoid biases and inaccuracies
Monitoring and tracking data quality metrics
3. Content Customization and Personalization
Generative AI content can be tailored to suit specific audiences, industries, and formats. This customization is essential for enhancing user experience and driving engagement. Personalization can be achieved by:
Fine-tuning AI models to generate content for specific niches or industries
Adjusting language, tone, and style to cater to different audiences
Incorporating user preferences and behavioral data into the content generation process
4. The Human Touch in AI-Generated Content
Although AI-generated content can mimic human language, it may lack the creativity, emotion, and cultural understanding that comes naturally to human writers. To overcome this limitation, it's essential to involve human editors and subject matter experts in the content generation process. They can:
Review and refine AI-generated content for clarity, coherence, and relevance
Ensure that the content aligns with brand guidelines and messaging
Provide feedback to improve the AI model's performance over time
5. Content Distribution and Optimization
Once the AI-generated content is produced, it's essential to optimize its distribution. This involves analyzing performance data to identify which channels, formats, and publishing times yield the best results. Key optimization strategies include:
A/B testing different content variations
Adjusting content based on audience engagement data
Leveraging analytics tools to track performance and identify trends
Conclusion
Production management for generative AI content requires a combination of data management, content customization, human involvement, and optimization strategies. As AI-generated content continues to grow in popularity, staying ahead of the curve by mastering these techniques will be crucial for content creators and marketers alike. Embracing the potential of AI and adopting best practices for production management can yield significant benefits in efficiency, scalability, and content quality.
コメント